4 Ways To Achieve Reproducible Flow Cytometry Results
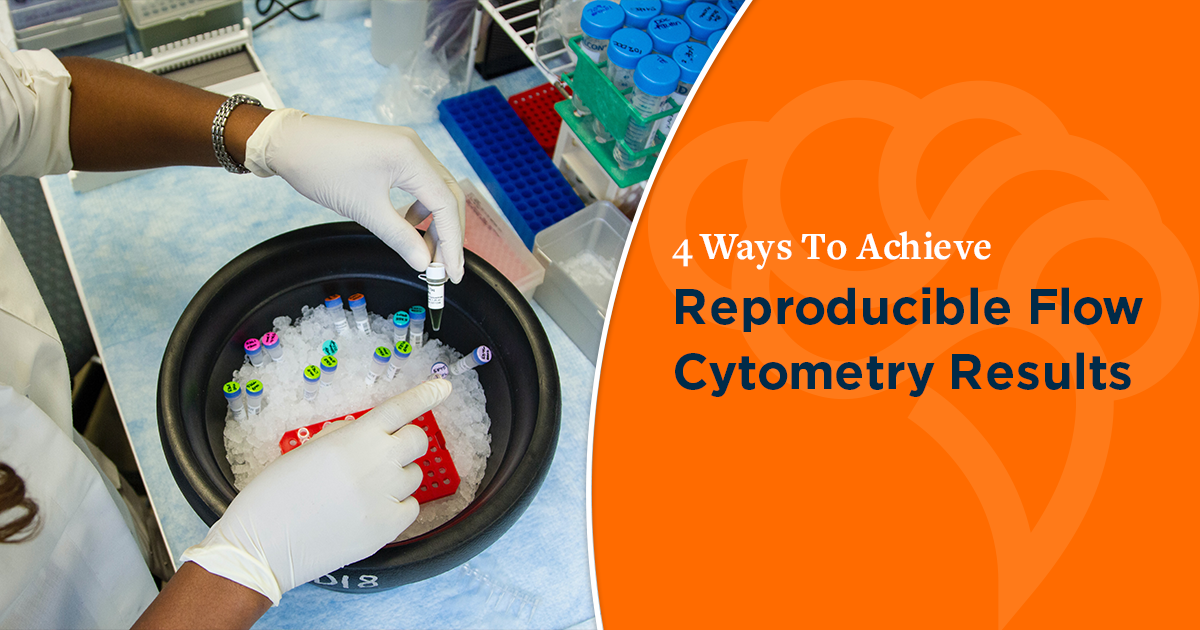
Reproducibility is one of the hallmarks of the Scientific Method. One reason scientists publish their results is so that other labs can attempt to reproduce the results and extend the investigation into new areas.
From the Begley and Ellis commentary in Nature, to the development of the Rigor and Reproducibility initiative at NIH, to articles in the mainstream media, reproducibility is on everyone’s mind. This is not a bad thing, and following the best practices in flow cytometry provides investigators, peer reviewers, and colleagues with more confidence in your data.
There are several areas within the process of developing, implementing, and reporting a flow cytometry experiment where a little additional work, attention to proper controls, and careful planning will ensure reproducible data generation.
Instrumentation: Characterization, Optimization, and Quality Control.
The first line of defense for good, reproducible data is the instrument.
A properly maintained machine ensures that it is not introducing error in the data. A researcher needs to know that when they find a difference, it’s due to the experiment, not the instrument. Thus, quality control is the key.
In the recent “Best Practices” article from the ISAC Shared Resource Laboratory Taskforce (Barksy et al. 2016), the authors discuss the importance of QA and QC, and highlight the different possible levels — including the idea of an external audit.
It is critical to remember that QC is only good if it is being monitored. Running beads without looking back at the trends over a week, a month, or a quarter doesn’t help anyone.
The end-user should not be shy about asking those in charge of the instrument to see the QC reports or Levey-Jennings plots (Figure 1).
The end-user can also take ownership of QC for their experiments by incorporating a bead standard that is run before each experiment in the series, and monitored. Such a practice was discussed in a recent paper by Misra and co-workers (2016).
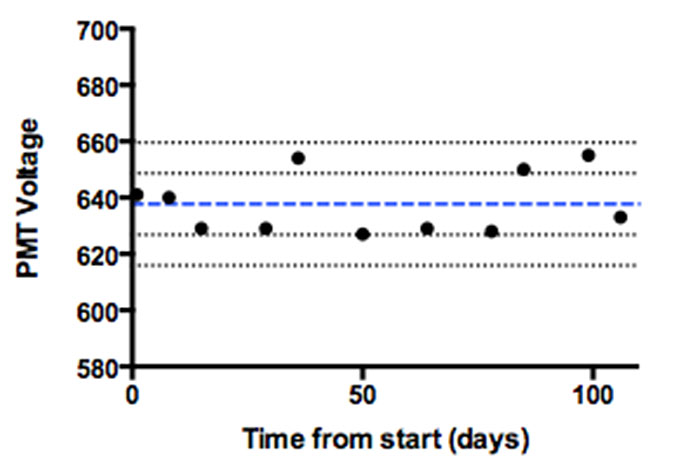
Figure 1. Levey-Jennings graph of QC data monitoring PMT voltage changes over a 100-day period. The blue line represents the mean, and the black lines +/- 1 and 2 standard deviations around the mean. Depending on the experimental needs, this data can be used to determine when an intervention is necessary before running a critical sample.
Another area that investigators should look for, regarding the instrument, is optimization of the system. At a minimum, the optimal PMT voltages and linear dynamic range should be available to all users. Additional characterization, such as the protocols discussed in this paper by Perfetto and co-workers (2012), can help with panel design.
It may seem like a lot of work, but what is the cost of a ruined experiment? Lost data? It is critical that researchers begin to ask for this level of characterization from their instruments, and that those operating them are given the time and resources necessary to perform such characterizations.
Reagents: Cells, Antibodies, and Buffers.
In many cases, our flow cytometry experiments will be measuring a biological process in cells and cell lines using fluorescently tagged antibodies.
The issue of cross-contamination of cell lines is well-documented, and it is incumbent on the investigator using cell lines to provide validation of the cell line in question. With advances in next generation sequencing and proliferation of commercial services to perform this validation, it is critical that you know the cell line you are using.
A more deeply-rooted problem may be naming conventions. In a recent Nature article, Yu and colleagues suggested a new framework for naming cell lines.
Antibody naming conventions have benefited from the Human Leucocyte Differentiation Antigens (HLDA) workshops, which share information on their website about different antigens and antibody clones available for investigators.
Regarding antibodies, optimization of these reagents is an essential component to good flow cytometry. Titration is the first important step towards characterization of a given antibody. Performing this assay allows for determining the best concentration for your experimental assays, as well as validating that the antibody is working.
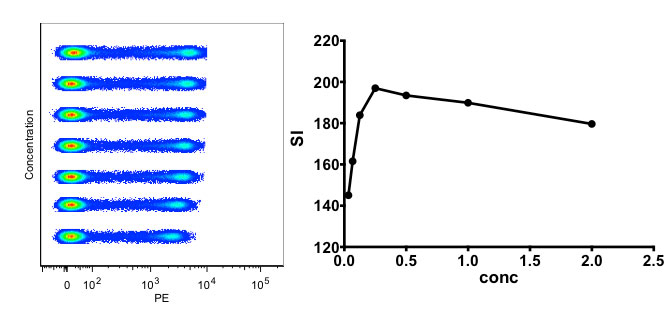
Figure 2. Titration of an antibody. On the left is the concatenated file with increasing antibody concentration, and on the right is the calculated Staining Index calculated per Maecker et al. (2004).
Additional validation steps of the antibody is a critical discussion that is ongoing in the literature.
While we currently rely on vendors to ensure their processes provide us with what the label says, a recent letter in Nature by Bradbury and Plückthun (and 110 co-signers) highlighted our dependence on protein binding reagents and their current limitations, as well as the magnitude of research dollars lost to poor quality reagents. It will bear watching to see how the industry moves in the directions that this letter advocates.
A quick comment on buffers and reagents — many investigators rely on purchasing pre-mixed reagents as a way to improve consistency and lot-to-lot variation over house-made reagents. It is important to ensure that you have a proper training and validation protocol for reagents, regardless of their origin, to make sure that they are not negatively affecting your data.
A cautionary tale on using pre-made reagents is found in this paper by Zarkowsky and co-workers (2011). Here, the researchers noticed a loss of quantum dot fluorescence with some lots of commercial formalin solutions.
After characterization of different lots, it was discovered that copper ions, a micro-contaminant, were the culprits (Figure 3). The solution to the problem was simply to add EDTA to the fixative, which chelated the Cu++ ions.
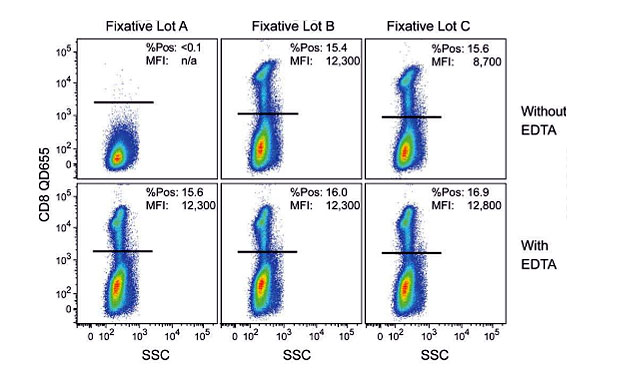
Figure 3. Figure 1 from Zarkowsky’s paper, showing the effect on Qdot fluorescence of three different lots of fixation buffer (+/- EDTA).
One can only imagine how many investigators lost data between the adoption of quantum dots in flow cytometry and the publication of this paper. It serves as a cautionary tale for researchers adopting newly available fluorochromes to delve a little deeper into their buffers in the case of anomalous results.
Process: Monitoring Human Error.
Variability in data can be a result of sample processing. There are many steps between isolating the cells and acquiring data. Development and use of standard operating procedures (SOPs) can help mitigate variations. A mainstay of clinical labs and GMP production facilities, more and more basic research labs are beginning to adopt SOPs. The aforementioned Barksy et al. 2016 paper spends a good deal of time talking about their role in flow cytometry.
Another way to monitor the sample preparation process is to develop and validate a “reference” or “process” control. This is a standard sample that is run each time the assay is performed.
Knowing how this standard sample performs in the assay will allow the researcher to set acceptable limits and know if there was an issue with part of the process (Figure 4). The severity of the deviation determines whether it is possible to troubleshoot and move the assay forward, or if the run has to be performed again. Likewise, it can point to instrument issues that developed between the QC and the time the investigator ran the assay. Of course, as with any QC system, it must be monitored.
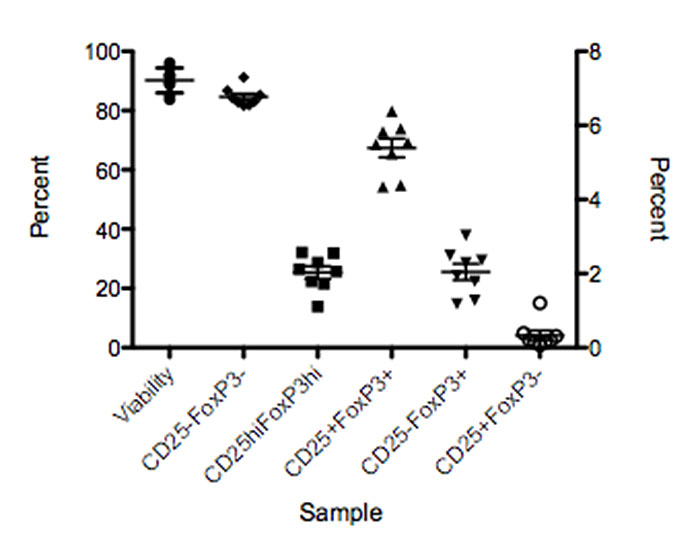
Figure 4. Process control sample showing the range of expression for different populations in an assay.
Analysis: Extracting the Correct Data.
The fourth area for consideration to improve reproducibility of flow cytometry results is data analysis. This is the area where the ‘science’ of cytometry meets the ‘art’ of analysis, and the one that causes the most headaches.
At present, most flow cytometry analysis relies on manual bi-variant hierarchical gating strategies. As more robust automated workflows are developed, they may become the dominant analysis methods, reducing or removing human bias from the process. Until that time, however, a few simple rules can help improve analysis and reduce errors.
Before analysis begins, it is important to understand what the biological question is, what data needs to be extracted from the experiment, and what downstream analysis will be performed on the data. This will drive the development of the analysis strategy.
To develop a robust analysis strategy, one must understand best practices for gate setting. This is the basis of a separate blog post, as there are many factors involved in that process.
Good analysis requires using appropriate controls, understanding display options, and developing consistent rules, once again leading to the SOP.
In the recent update to FCS Express, they included an SOP builder in the software. This allows for the creation of an analysis SOPs for lab members to follow. Checkpoints can be built in throughout the analysis, so that the researcher moves through step-by-step, and will not proceed to the next step unless the requirements are met. It’s a great feature for a lab performing a standard analysis over time, so that everyone performs the analysis the same way.
Consistent analysis is critical, as was shown in a study published by Maecker and coworkers (2010), which compared the analysis of prestained cells in 15 experienced labs versus analysis in one central lab. The results showed a mean CV of 20.5% across the four samples for analysis by the remote labs, versus 4% when the analysis was performed in a central lab. That level of data spread can make it extremely difficult to find rare differences, thus reducing the likelihood of advancing the field.
For consistency in gating, relying on more normal shapes for gates (rather than free-form drawings) is an important consideration (Figure 5). Couple that with the use of FMO controls, establishing cut-off percentages for gates, and acquiring appropriate biological controls, one can improve the consistency of results.
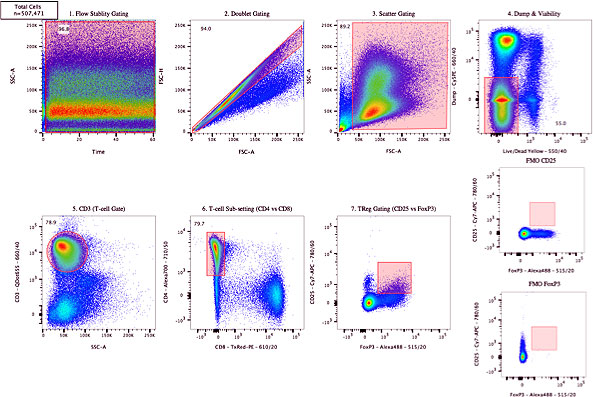
Figure 5. Sample analysis showing a gating strategy for analysis of a multi-year study.
Finally, because there is a great deal of information that cannot makes its way into a standard materials and methods section, the ISAC Data Standards Task Force developed and published the “Minimal Information about a Flow Cytometry Experiment” or MIFLowCyt standard. This standard establishes a detailed checklist covering four major areas:
- Experimental overview
- Sample and specimen description
- Instrument details
- Data analysis details
Papers published that are compliant with this standard ensure an additional level of information is available to researchers seeking to reproduce the data.
At present, this standard has not been widely adopted, but is strongly encouraged for submissions to Cytometry A. A checklist is available from the journal to assist in ensuring a submitted paper has met the reporting requirements of this standard.
Additionally, ISAC has developed and supports the FlowRepository, a database where investigators can submit their data. This database, first described in a paper by Spidlen and coworkers, allows peer reviewers access to the raw data used in a paper so they can examine the data to improve the review process.
After publication, the data is available to investigators as a way for them to compare their results with published data.
An excellent example of the FlowRepository in action are the Optimized Multicolor Immunofluorescence Panels (OMIPS). These papers describe the development of new polychromatic flow cytometry panels for the research community, and are required to show the gating strategy used by the authors. Having the primary data available to the research community again offers a way to combat issues in reproducibility.
In conclusion, there are several areas that researchers can focus on to improve the reproducibility of their flow cytometry experiments. From instrument quality control, through validation of reagents, to reporting out the findings, a little effort will go a long way to ensure that flow cytometry data is robust, reproducible, and accurately reported to the greater scientific community. Initiatives by ISAC have further offered additional levels of standards to support these initiatives, which were developed even before the Reproducibility Crisis came to a head in both scientific and popular literature.
As scientists, we owe it to ourselves, our colleagues, and the public to ensure the data we generate is of the highest quality. It is Isaac Newton who is attributed to saying, “If I have seen further, it is by standing on the shoulders of giants.” Together, following the steps outlined above, we can each stand on the shoulders of our colleagues to move scientific discovery forward, with the ultimate goal of improving the health and well-being of our fellow man.
To learn more about 4 Ways To Achieve Reproducible Flow Cytometry Results, and to get access to all of our advanced materials including 20 training videos, presentations, workbooks, and private group membership, get on the Flow Cytometry Mastery Class wait list.
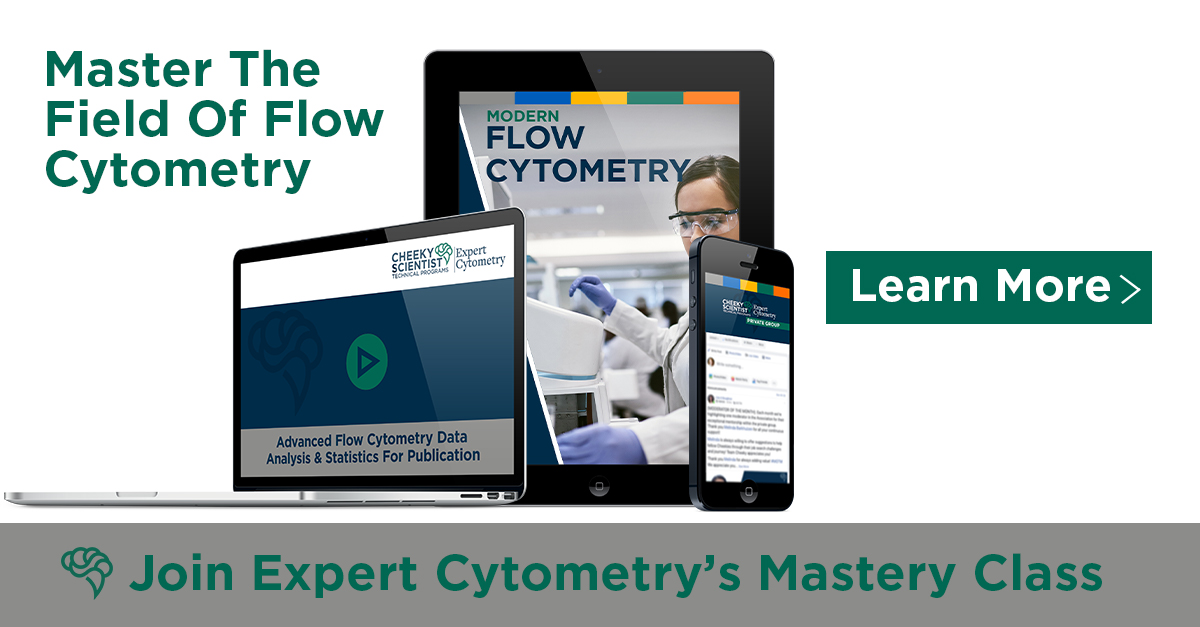
ABOUT TIM BUSHNELL, PHD
Tim Bushnell holds a PhD in Biology from the Rensselaer Polytechnic Institute. He is a co-founder of—and didactic mind behind—ExCyte, the world’s leading flow cytometry training company, which organization boasts a veritable library of in-the-lab resources on sequencing, microscopy, and related topics in the life sciences.
More Written by Tim Bushnell, PhD