2 Key SPADE Parameters To Adjust For Best Flow Cytometry Results
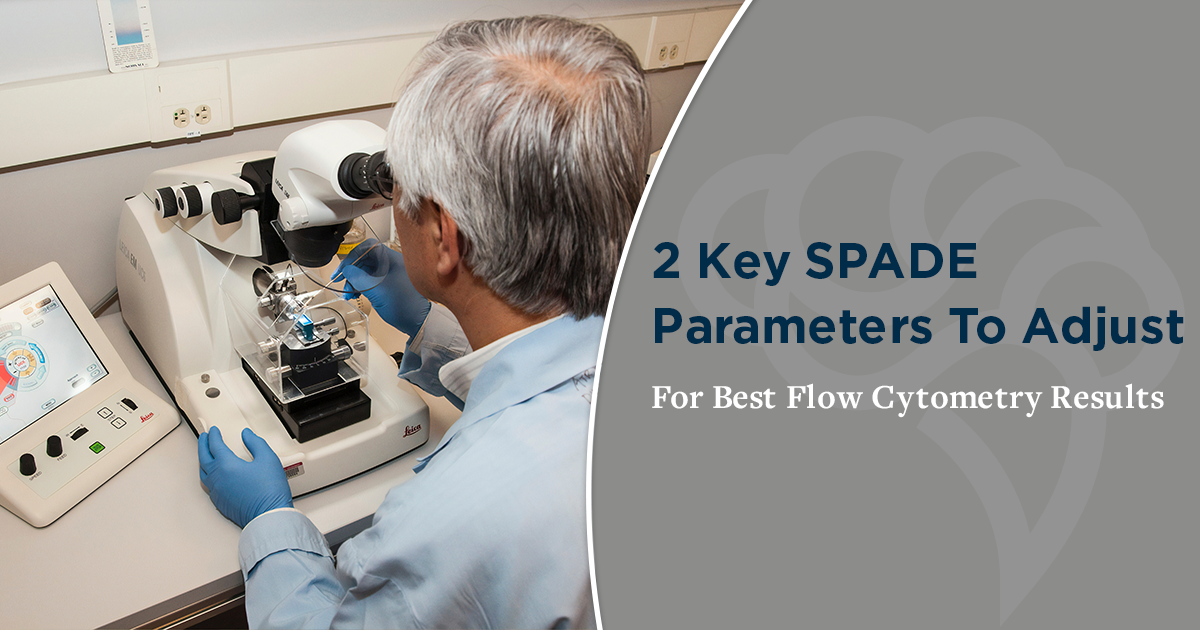
Mass cytometry panels routinely include 30 or more markers, but traditional analysis methods like bivariate gating can’t adequately parse the resulting high-dimensional data.
Spanning-tree progression analysis of density-normalized events (SPADE) is one of the most commonly used computational tools for visualizing and interpreting data sets from mass cytometry and multidimensional fluorescence flow cytometry experiments.
There are two key parameters in SPADE that you can adjust in order get the best results possible: downsampling and target number of nodes, or k. Knowing how to properly set these values will enable you to enhance the quality of your analysis.
Downsampling
Imagine your data as a cloud of points in high dimensional space, where each dimension is one of the measured markers.
Cells that are similar to each other are close to one another in this cloud, just as similar cells fall together on a biaxial gating plot. This means that the cloud contains dense regions where there are groups of similar cells, and more sparsely populated regions where there are few similar cells.
The cells falling around the edges of dense regions will likely be grouped into the larger clusters during analysis, even if some of the sparse regions contain cell subsets that happen to be small but phenotypically distinct.
Downsampling in the SPADE algorithm reduces the density variation across the cloud in order to give more equal weight to small, less dense groups of cells in the clustering process so they won’t get absorbed into the larger, denser regions.
After downsampling, SPADE clusters the data and then upsamples, in order to map the cells that were removed during downsampling, back into the clusters to which they are most similar.
You can adjust the extent of downsampling by changing the percentage or absolute number. The percentage indicates the percentage of cells you want to keep during the downsampling process.
100% downsampling means that 100% of the cells will be kept, and therefore SPADE will not downsample. 5% downsampling means that only 5% of the cells will be kept for clustering. Lowering the downsampling percentage in this way prevents small or rare populations of cells from being lost in the clustering step.
If you set an absolute number, rather than a percentage, SPADE downsamples until this number of cells remains.
If you’re working with a limited number of relatively large populations, like normal blood cells, you can probably safely leave the downsampling percentage set to the default. However, if you are seeking novel populations of cells, or very small populations like stem cells, you should consider setting the downsampling to a lower percentage in order to prevent losing those populations during clustering (Figure 1).
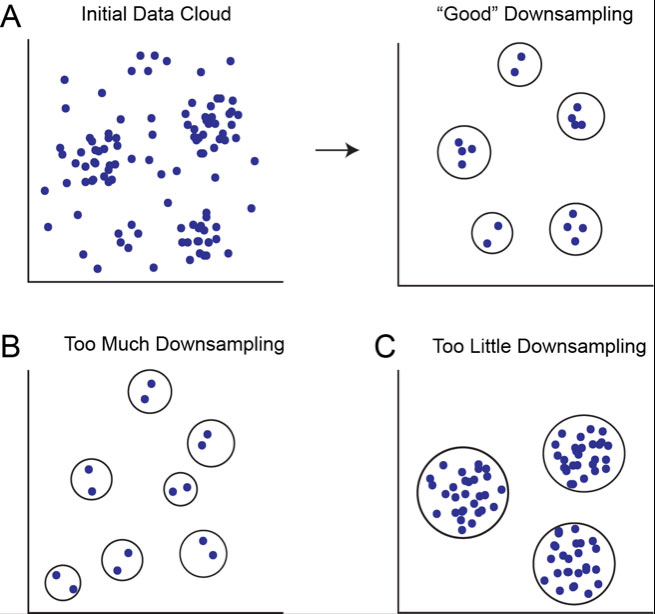
Figure 1. Downsampling removes density variation to determine which regions of the point cloud constitute discrete clusters. A) Initial data cloud in n dimensions shown before and after appropriate downsampling, assuming five real cell populations in the data. B) Clusters determined after too much downsampling. Low density regions are inappropriately considered to be discrete clusters. C) Clusters determined after too little sampling. Only high density regions are considered to be clusters.
An important consideration is that when you set the downsampling percentage very low, you risk focusing on noise in the data. Sparse regions in the high dimensional cloud might be treated as discrete clusters, when in reality, they represent nothing more than noise.
On the other hand, if you set the downsampling percentage too high, or if you don’t downsample at all, you risk overlooking smaller, “real” populations of cells.
Target Number of Nodes (k)
The second parameter that you can adjust in a SPADE analysis is the target number of nodes, or k. This value indicates the number of populations into which you want SPADE to divide the cells.
Keep in mind that this number is a target, not an exact value, so you may notice empty clusters in the final output if SPADE couldn’t find exactly k number of clusters in the data.
A good rule of thumb is to always ask SPADE for more nodes, or clusters, than you expect to find.
Overclustering in this way allows you to identify potentially unexpected subpopulations that are defined by subtle, high-dimensional patterns of marker co-expression (for example, small subpopulations of T cells in normal blood that are defined by subtle differences in their co-expression of several activation markers).
Additionally, overclustering helps you delineate between major populations because when the SPADE tree is populated by more nodes, it is easier to visualize and determine more precisely where one major population, or group of nodes, ends and another begins.
When choosing k, you should consider how many populations you expect to find, the relative size of those populations, and the total number of cells in the data set.
If you ask SPADE for 500 clusters but only have 1,000 cells and 5 major populations, you’ll probably get back lots of empty clusters as well as clusters with only a few cells each.
It’s crucial to consider the biological implications of what you put into SPADE, and what you get back. For example, is a population of T cells that only has 3 out of the 1,000 cells “real” or significant?
Knowing your data and your biological system can help you decide appropriate cut-offs for k values, as well as what population sizes are likely to be biologically valid, versus just noise.
Another consideration is that small subsets resulting from high cluster numbers can also be more unstable, meaning that the cells’ phenotypic similarity is so subtle that another round of clustering might group them differently, and thus you may find that these populations won’t hold up to further computational or experimental scrutiny.
Conversely, setting k too low can cause you to miss smaller populations, as they’re likely to be merged into the larger, denser clusters of cells (Figure 2).
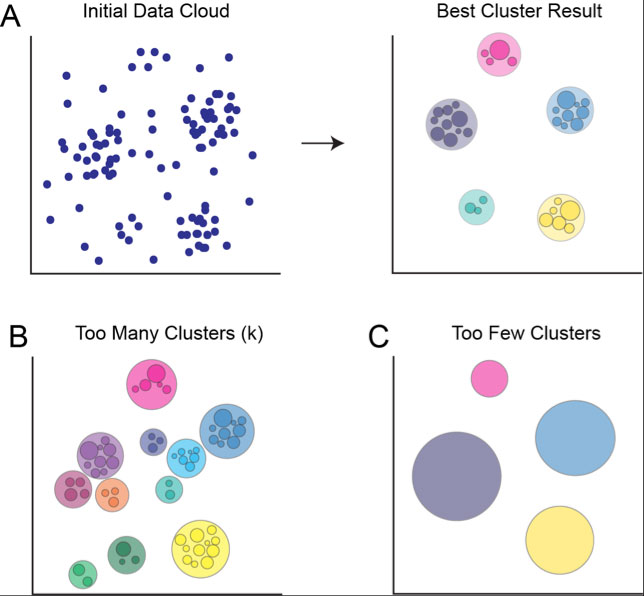
Figure 2. Target number of nodes (k) affects the number of clusters returned by SPADE. A) Initial data point cloud before and after clustering using appropriate target number of nodes, assuming five real populations of cells in the data. Data is properly overclustered, allowing analyst to manually delineate between major populations. B) Clustering result if target number of nodes is too high. Major populations appear to contain multiple clusters, and lower density regions are designated as discrete clusters, containing very few cells. C) Clustering result if target number of nodes is too low. All cells are grouped into a few large clusters, obscuring smaller populations of cells.
Fine-tuning your SPADE analysis requires a k value and downsampling percentage that will identify small, rare cell populations without blowing out noise in the data.
Going forward with your analysis, it’s always crucial to experimentally validate novel populations that you have discovered, using SPADE or other computational methods.
For further reading, see: Qui, et al. Extracting a cellular hierarchy from high-dimensional cytometry data with SPADE. Nature Biotechnology, 2011.
To learn more about 2 Key SPADE Parameters To Adjust For Best Flow Cytometry Results, and to get access to all of our advanced materials including 20 training videos, presentations, workbooks, and private group membership, get on the Flow Cytometry Mastery Class wait list.
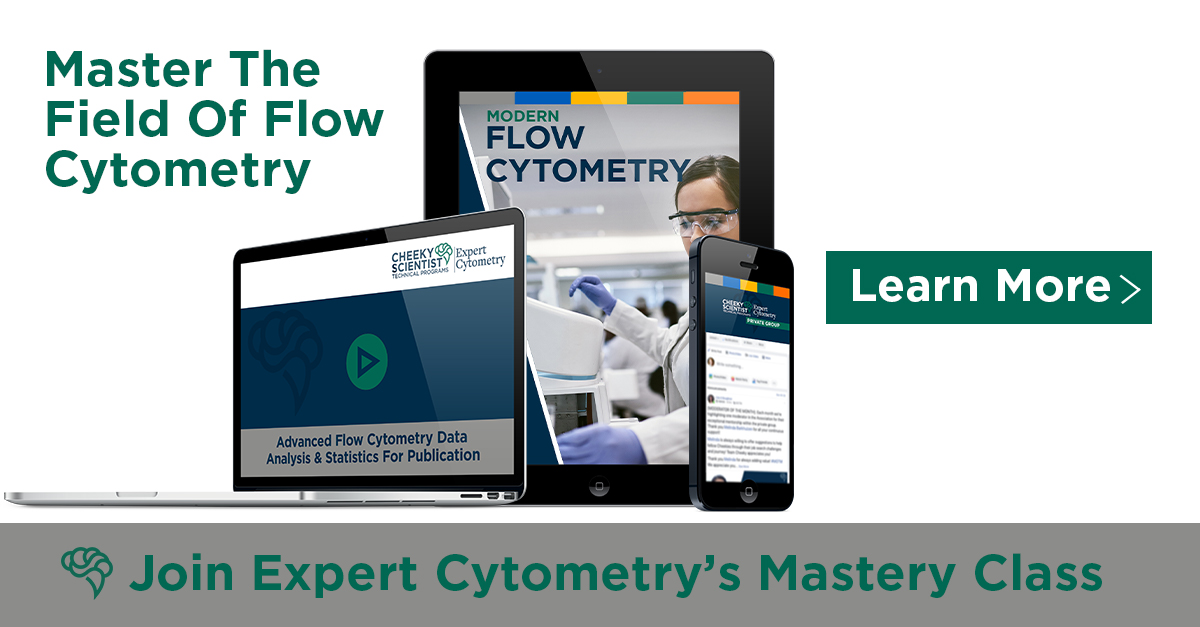
ABOUT TIM BUSHNELL, PHD
Tim Bushnell holds a PhD in Biology from the Rensselaer Polytechnic Institute. He is a co-founder of—and didactic mind behind—ExCyte, the world’s leading flow cytometry training company, which organization boasts a veritable library of in-the-lab resources on sequencing, microscopy, and related topics in the life sciences.
More Written by Tim Bushnell, PhD