The Essential Dos and Don’ts of NGS (Next Generation Sequencing)
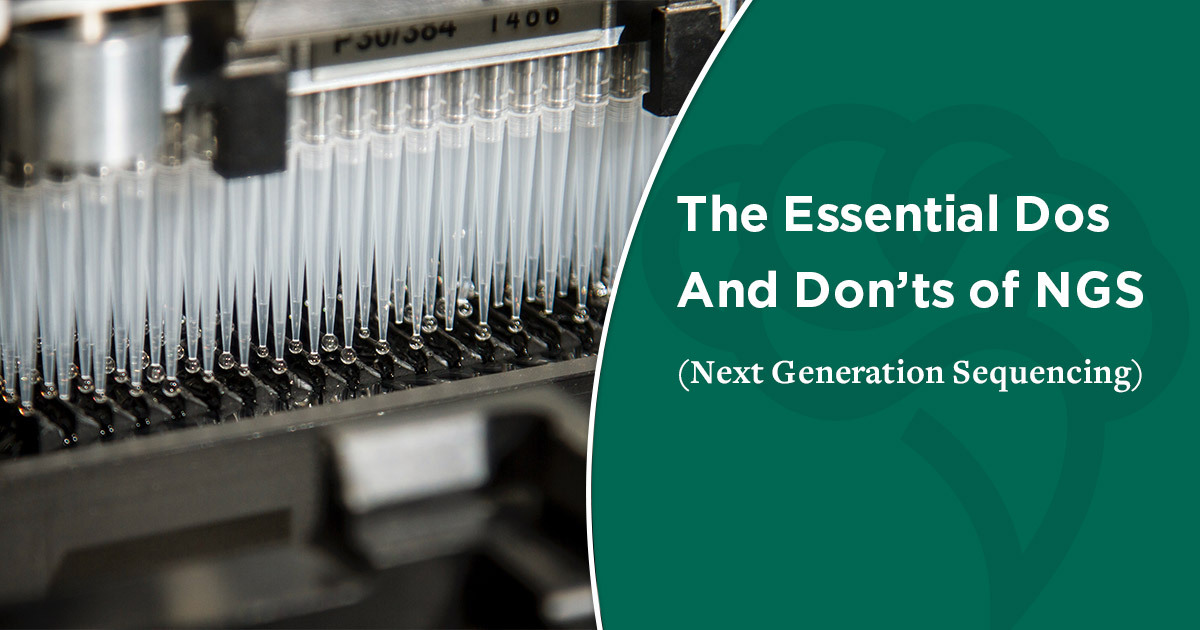
Next Generation Sequencing (NGS) is a rapidly evolving and widely used method worldwide in both academic and non-academic settings. One of the most valuable aspects of NGS is producing millions of sequenced reads with diverse read lengths from small amounts of input DNA. NGS methods are extremely versatile; producing reads as short as 75 bp, as seen in SOLiD sequencing, to long reads ranging upwards of 1000bp in the case of Pyrosequencing.
Both long and short reads fill a unique niche for researchers. Longer reads generated from NGS are excellent for genomic rearrangement and genome assembly projects; especially when there is no reference genome available. Shorter reads prove substantial to fill in the gaps in the generated assemblies, such as scaffolds.
With its significant impact in clinical applications in determining pathogenic and deleterious mutations in diseases, NGS is on the forefront in helping pathologists and researchers in making accurate diagnoses. As we can fathom, NGS is beyond just generating sequenced read data – it’s the platform where researchers can delve deeper into determining the hidden information within genomes. All this information and immense amount of data can be used to find answers to some of the biggest clinical questions.
However, there are many limitations and challenges to this technique. NGS requires a highly skilled team of professionals, bridging together knowledge from integrative bioinformatics, computational biology and clinical research. With the significant impact of NGS in healthcare, it is imperative to implement safe and high quality NGS practices during DNA sampling and library preparation. Researchers should keep a strict watch on the Dos and Dont’s of NGS in order to ensure they are conducting the highest quality NGS experiments and thus producing significantly accurate results.
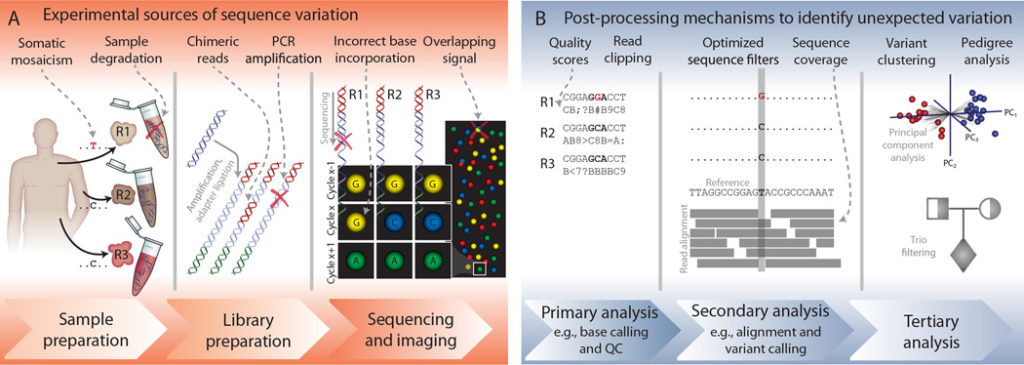
Sample Preparation Stage Errors
Sample preparation is the first step in the process and should be done carefully. Foolish sample preparation can lead to cross-contamination and sample degradation between samples. For instance, samples can be heterogeneous, containing multiple clones of tumor cells, or inherently contain DNA from different species. The best way to avoid cross-contamination during sample preparation is sterilization. Always sterilize your workbench and all sample preparation tools such as scalpel blades and pipettes.
Moreover, utmost care should be taken in handling different samples – in that regard, it is the best practice to handle one sample at a time. Additionally, you should include DNA-free samples along with actual samples as a quality control step in detecting cross-contamination. Obviously, these DNA-free samples should not be used for sequencing.
The other “do” in sample preparation is: have an adequate amount of high-quality DNA. This is pertinent in diminishing PCR amplification errors and in increasing sequencing read depth. Thus, you should design experiments ensuring that ample DNA template material is available for sequencing. However, in NGS, the threshold of required input DNA is not high (for most applications, at least 200 – 500 ng of total DNA template at the minimum required).
Contamination within the sample is also quite common and should be avoided if possible. However it’s not always easy to avoid – such as somatic DNA in a tumor sample. This contamination can be addressed by ensuring that the input DNA is predominant. As a result, exogenous DNA can be filtered during the analysis phases.
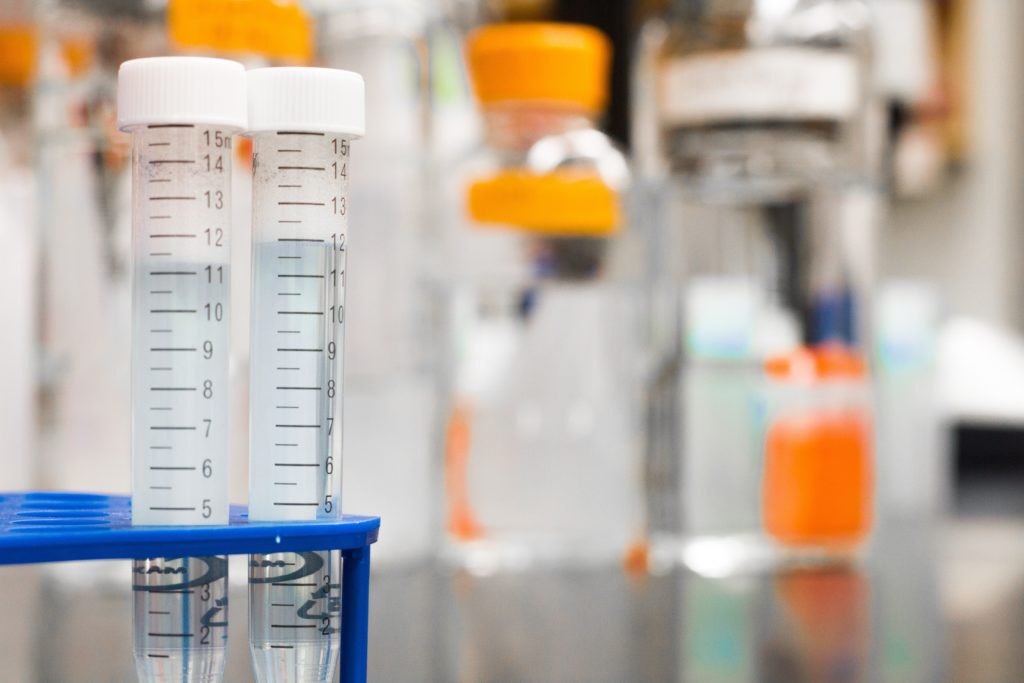
DNA Library Preparation Stage Errors
Primer issues can introduce errors during DNA library preparation. Primer binding bias, mispriming, non-specific binding, primer-dimer, hairpins, or chimeric reads formation are some of the errors that may arise during the library preparation phase. Special attention should be paid to avoid chimeric reads formation during amplification since these reads mimic biological sequences to a great extent and hence are very difficult to be trimmed from the sequenced read data.
Library complexity can be another source of significant errors in NGS. Library complexity signifies the representativeness of the DNA library of the actual sample. There are a number of ways to ensure that the library complexity is properly assessed. Here are just a few:
- Use a probe-specific single molecule molecular inversion probe (smMIP) method, coupled with targeted NGS to quantify low-frequency targets.
- Use a small-scale targeted sequencing reaction or sequencing at lower depths to observe read distribution. This method could be useful in determining the coverage uniformity. Coverage uniformity is a measure to see that the number of sequenced reads generated from sequencing method(s) are uniform, thus helping in providing accurate estimation of aberrations/mutations existence with ample coverage or sequencing depth.
- You can also calculate the percentage of duplicate reads vs unique reads during the analysis step, as duplicate reads increase with the increase in the sequencing depth.
Finally, batch effect is the systematic bias that arises from the molecular biology steps. Routine lab operations such as reagent batches, pipetting errors, different handlers, different sequencing runs and flow cells, can introduce batch effects. Minimizing batch effects can make a dramatic difference in the final result. Some ways you can minimize these effects are:
- Start with samples of similar quality and quantity
- Use/create master mixes of reagents whenever possible
- Use high fidelity polymerases to minimize PCR biases due to GC content, e.g. AccuPrime Taq
- Add a 3-minute denaturation step with subsequent PCR melt cycles extended to 80ºC . This will reduce amplification bias from high-GC targets
- The fewer amplification cycles you use the better
- Amplify every sample with the same number of cycles
- Pool barcoded samples in equimolar amounts before gel or bead purification
Sequencing Stage Errors
The errors coming from sequencers during the process of sequencing are difficult to control since they are machine-specific. For instance, Ion-torrent technologies may fare poorly with repetitive stretches of the same nucleotides. These errors can be mitigated by ligation-based SOLiD NGS method, but SOLiD produces much shorter reads that could present problems during the computational analysis steps. Hence, it is pertinent to establish a good understanding of the factors that may introduce sequencing errors. Here are some of the top factors that contribute to sequencing stage errors:
- Cross-contamination between flow cells
- Dephasing, such as incomplete extension or addition of multiple nucleotides per cycle
- Degradation of fluorophores or nucleotide reagents, and overlapping signals
- GC-biases, repeats, and low-complexity regions within the sample
- Reads being omitted due to difficult to sequence regions
- Strand bias
- Problems with the machine itself
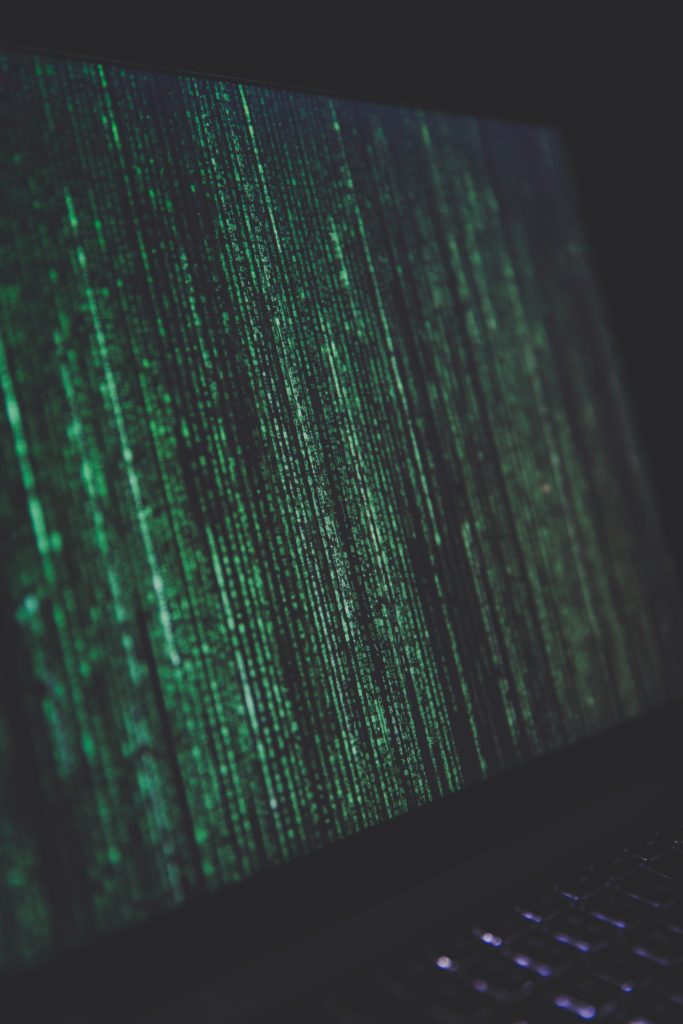
Dos To Improve The Efficacy of NGS Experimental Procedures
You can perform Pilot Runs using known samples to test the NGS protocols and facility setup. During this step, the entire pipeline from sample harvesting to DNA extraction, library preparation, sequencing, and analysis steps is tested. This phase can prove to be useful in designing quality control measures, efficiently estimating true and false positives, addressing potential library complexity issues, identifying the best sequencing depths, documenting timelines, and testing for machine performance.
Replicating experiments is a great way to minimize errors due to batch effects, identify other sources of variations, and measure the efficacies of bioinformatics analysis pipelines. There are three different kinds of experimental replication:
- Technical: repeats of the same analysis of the same sample.
- Biological: analysis of multiple samples or multiple samples from the repeats of the same conditions.
- Cross-platform: analysis of same sample(s) on multiple NGS platforms to mitigate platform-specific sequencing errors.
Randomization of samples can help to reduce biases caused due to batch effects, instrument effects, systemic biases, and other confounding factors, by addressing independent variables that are not already accounted for in the experimental design. For example, it is a good practice to randomize samples with different adaptor sequences, and multiplex the samples along with controls.
Orthogonal method validation can be used to mitigate inherent errors from the experimental processes and sequencing technologies. Some examples are: PCR genotyping, Sanger sequencing, and restriction fragment length polymorphism (RFLP) analysis; however, it is recommended to use both positive and negative controls for these validation steps.
Post-Processing Steps to Identify False Positives
Eventually, by following the above measures and Dos and Don’ts, researchers can eliminate errors caused by experimental sources. However, you should understand that no measure and care during experimental procedures can give a hundred percent accuracy in generating error-free sequenced reads. Hence, there are post-processing mechanisms to identify false positives (type I error) and other unexpected mutations in the sequenced data.
The one parameter that holds significance in determining the quality of the identified bases during sequencing is the Phred Score outlined by Ewing et al. With the help of Phred scores, sequenced reads with poor quality can be eliminated; improving the quality of the sequenced data for further downstream secondary and tertiary analyses.
Nevertheless, relying on computational analysis methods to mitigate sequencing errors can lead to precarious and false negative (type II error) results. Subsequently, as we can acknowledge, researchers should put paramount efforts in assuring the efficacy of the experimental procedures in producing least error-prone data for sequencing; which eventually, could corroborate with computational methods in performing efficient primary, secondary, and tertiary analyses leading to accurate identification of variants and also elimination of type I and type II errors.
In the real world, performing error-free NGS experiments and computational analyses of the sequenced data is not feasible. However, by keeping the necessary dos and don’ts in mind while implementing these analyses, we can certainly minimize the occurrence of these errors and considerably improve the accuracy of the results.
To learn more about NGS, and to get access to all of our advanced materials including training videos, presentations, workbooks, and private group membership, get on the Expert Sequencing wait list.
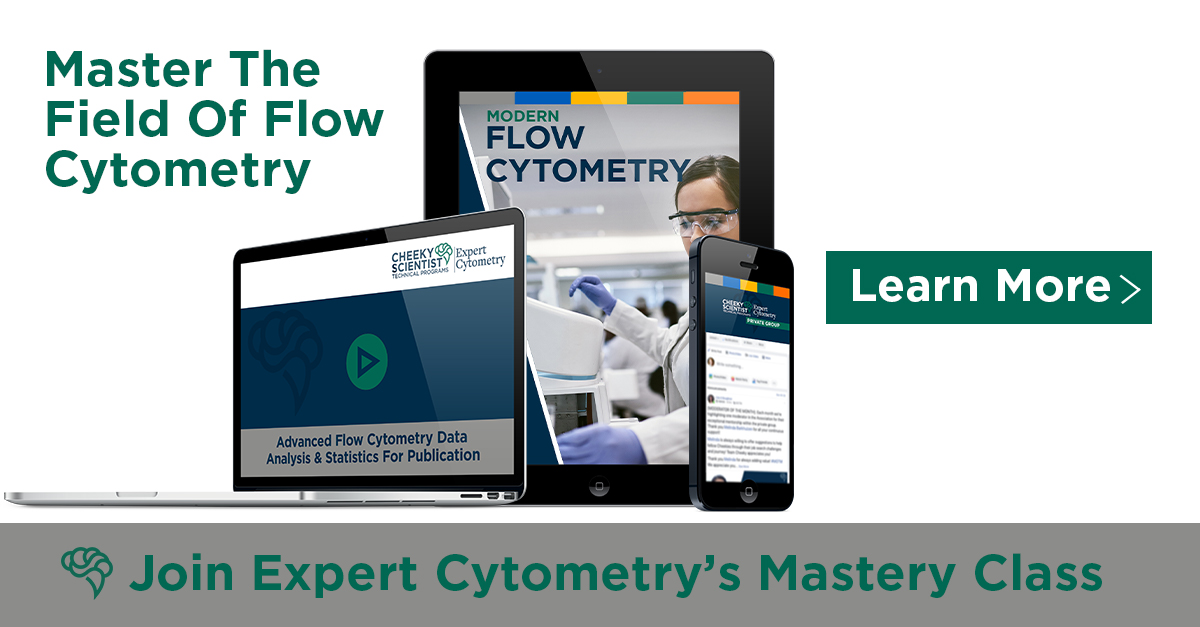
ABOUT DEEPAK KUMAR, PHD
GENOMICS SOFTWARE APPLICATION ENGINEER
Deepak Kumar is a Genomics Software Application Engineer (Bioinformatics) at Agilent Technologies. He is the founder of the Expert Sequencing Program (ExSeq) at Cheeky Scientist. The ExSeq program provides a holistic understanding of the Next Generation Sequencing (NGS) field - its intricate concepts, and insights on sequenced data computational analyses. He holds diverse professional experience in Bioinformatics and computational biology and is always keen on formulating computational solutions to biological problems.
More Written by Deepak Kumar, PhD